“It’s very, very rare to have a dev team that doesn’t do what you tell them to do, but instead listens and comes back with “hey, why don’t we do it this way?” or “we went ahead and did this”. One, you feel you’re hiring, the other one feels like family.”
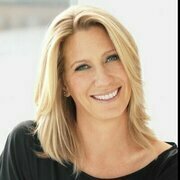
CEO & Founder
Client
Everywear is the brainchild of Brandon Holley, and it’s self-defined as the first B2B technology platform to bring editorial authority to e-commerce, through personalized recommendations. What does that mean? In their CEO & founder’s own words, it’s about “technology that thinks like a fashion editor”.
Everywear’s platform gives retailers the possibility to personalize the customer’s shopping experience through data-driven editorial expertise and deep data analytics, resulting in an ability to predict customers’ future purchase behavior, which is used to boost sales conversion.
Requirements & Context
Everywear was born out of Holley’s realization that the fashion magazines industry was dying, but that didn’t mean the customer’s emotional bond with the experience of shopping for clothes was too. So she decided to carry that feeling onto other media, and thus Everywear was born.
For her, it was clear that it’s much more motivating to shop alongside a friend with great taste, who can give advice in real time, in opposition to most e-commerce sites’ approach, which is based in the seller’s interest (what they need to sell) rather than the buyer’s (what they want to buy). Everywear intends to be that ‘friend’.
With that in mind, and after a couple of preliminary attempts, Manas was called in to give life to the platform that connects users with stylists to help them shop based on what's already in their closets.
Approach
Work began with regular weekly meetings between both teams, to get a feel for the most urgent issues to tackle, and to collaboratively design an efficient, feasible product. The goals for the project were: first, to make the infrastructure stable enough to keep the service running, and second, to optimize the architecture in order to reduce hosting services costs. With a solid, reliable infrastructure, development started on both front and backend.
The first stage of the project consisted of correcting live visualization for several of Everywear’s clients, and was completed in two months, in November 2018. The second stage consists of a series of demos of how the algorithm puts outfits together, based on parameters such as body type, occasion, and the customer’s basics (what’s already in their closets). And next on the roadmap is scalability. We are currently working on building the next-phase engine, powered with machine-learning and able to interoperate with different retailer setups and infrastructures.
Results
The algorithm is built on rules that Everywear’s CEO & founder used on-set for models and celebrities, but the entire platform hinges around the user experience: once the user is done listing all their “basics” (a.k.a. the contents of her closet, such as shoes, jeans, jackets, skirts, tops, pants and accessories like jewelry and handbags) onto the platform, Everywear then picks matching pieces of attire from the retailer’s catalog, and then displays outfits born out of combining items the user already owns, with those in the retailer’s product base. Using that input, the algorithm can instantly create several outfit options, for different occasions, like going to work, having dinner with friends or attending an evening event.
Items
Once their catalog is uploaded into the platform, retailers can select multiple items and tag them by season, style, bodytype, and others.
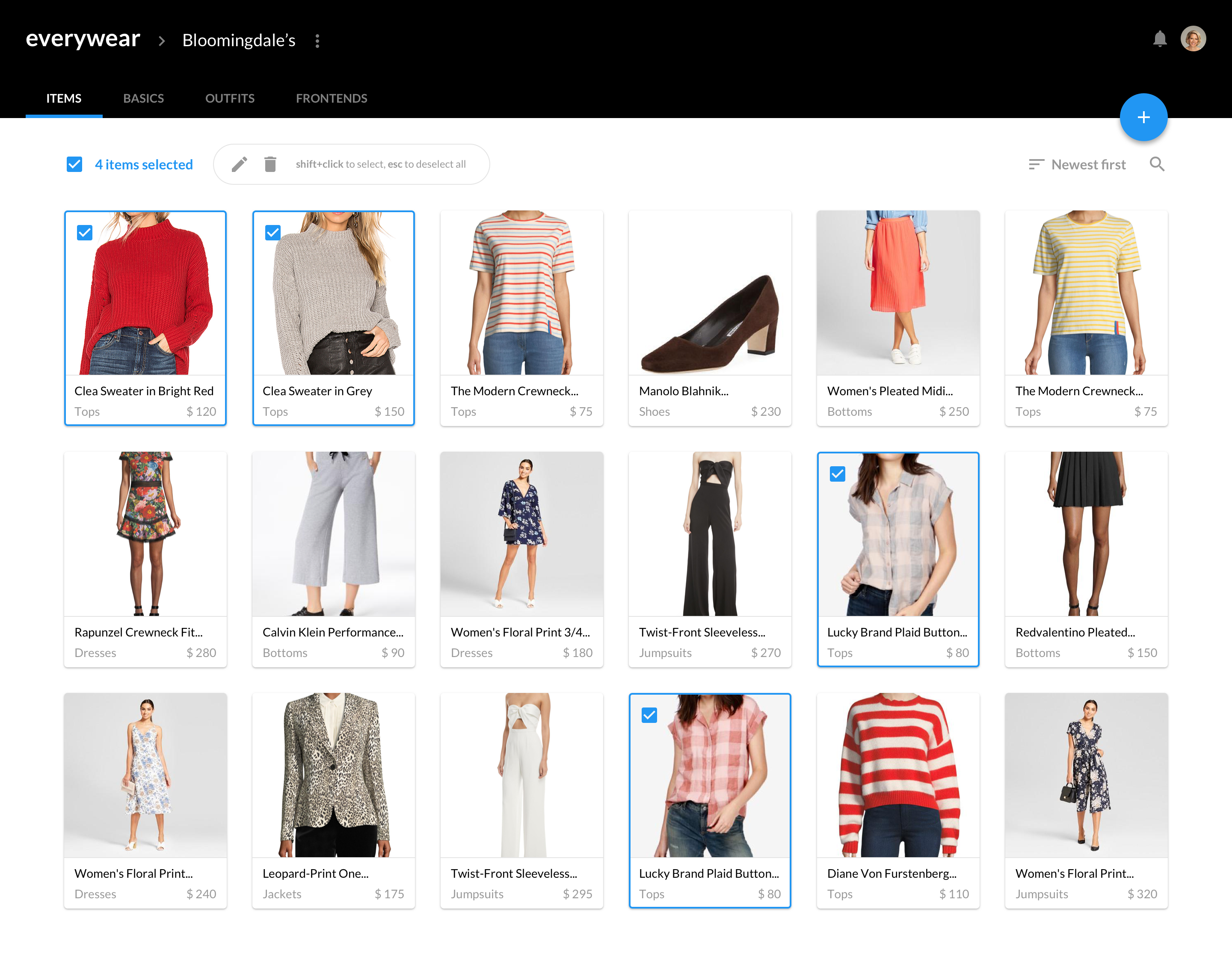
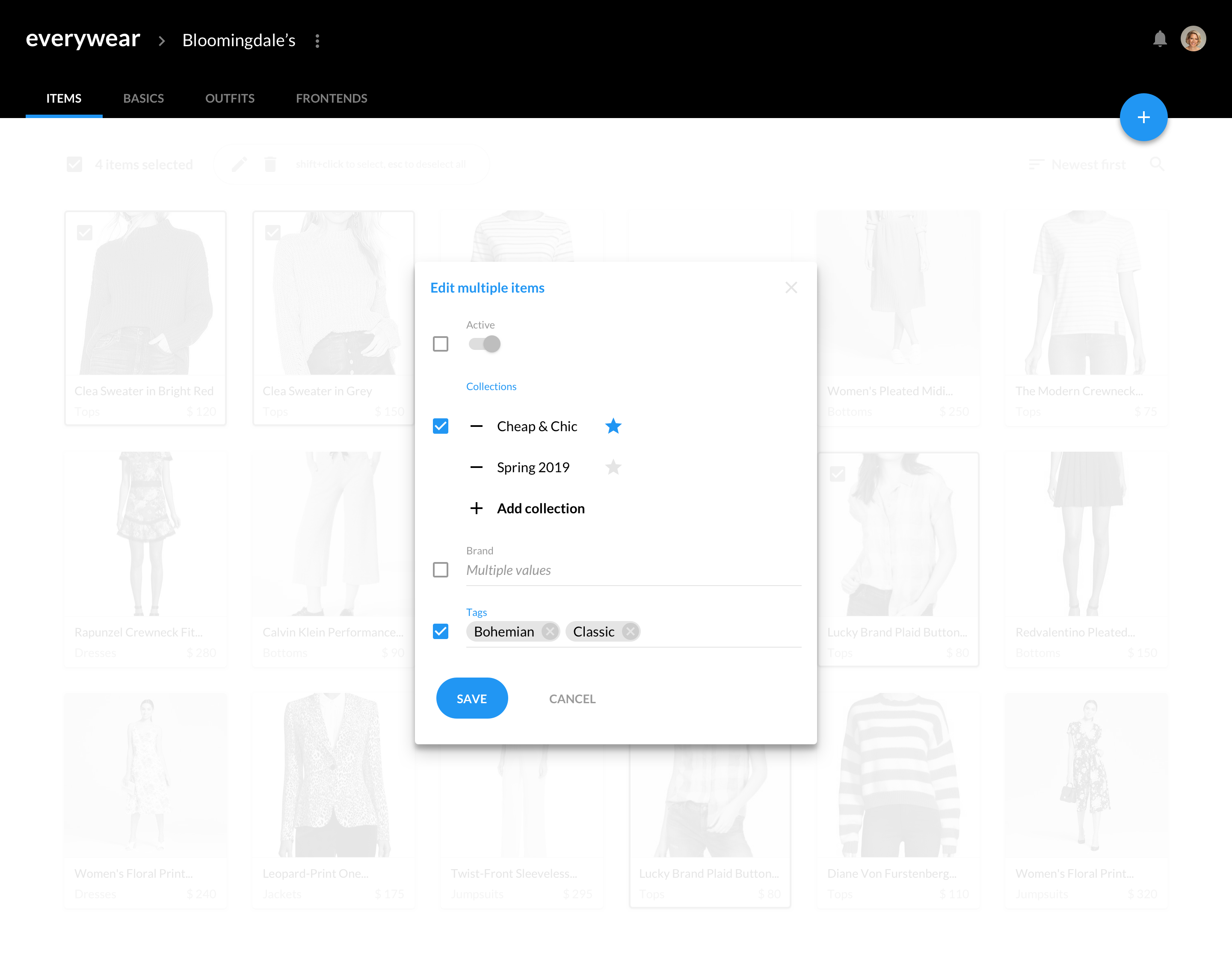
Item detail
Items can be tagged and described in detail, and multiple pictures can be uploaded into the platform.
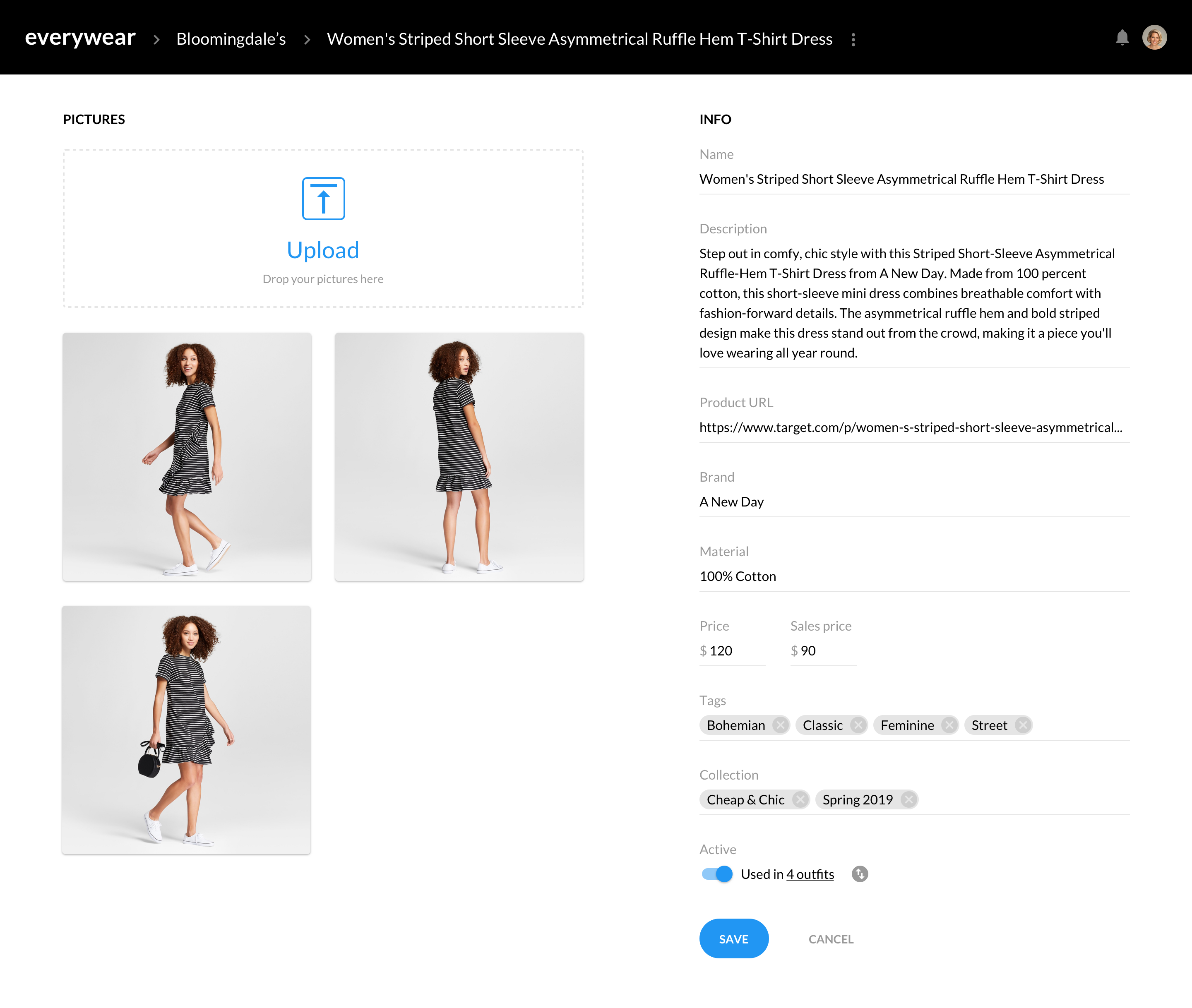
Impact
So far, Everywear has collected more than 2 million pieces of data from the closets of about 120,000 women who have used the platform. Those consumers have clicked on merchandise that’s worth, in total, almost $100 million, according to Holley.
Some tests have shown that Everywear increases the average sales-conversion rate up to five times, as compared to shoppers who didn’t access the platform, and the average order value was three times larger compared to shoppers who didn’t use the platform.
And also, the media picked up on it: