Concept
"Drones that can understand fire" sure sounds impressive, but it can come off as a little threatening. However, that is a fairly accurate way of describing ForestFires.tools: a set of tools that can forecast the spread of wildfires, almost in real-time, using UAVs, infrared imagery, machine learning classification of vegetation types, and a small portable weather station.
Requirements & Context
ForestFires was developed between November 2019 and February 2020, for the Unusual Solutions Competition, a global competition organized by WeRobotics, that invites individuals and teams from all over the planet to submit solutions on how to best tackle three relevant questions or challenges:
1. How can drone data and AI tools become more relevant to solving local social challenges?
2. How to turn drone data into action by bringing back drone data and insights to beneficiaries?
3. How can drones and the data collected using drones be used ethically to solve local problems?
The goal of the ForestFires.tools project is to aid fire responders in planning their efforts. Also, considering that many of the countries with the largest forest areas are in the global south, and are collectively less wealthy than their northern counterparts, we focused on putting together a solution that has a cost of around 600 USD, making it affordable to as many groups as possible. In future iterations, we plan to work with local UAV groups and maker-spaces in all countries, so that the product, training and support can be offered at an even lower cost.
Why it matters
Forest fires are sadly here to stay. Both in the northern hemisphere (in the Pacific Northwest and Siberia to name just a couple) but also in the southern hemisphere. Most recently, bushfires in Australia and the Amazon forest fires gained notoriety, but other areas like Central Africa and Patagonia have large forest fires every year.
These fires are destroying an extremely valuable natural resource: forests make up for around 30% of the Earth, and they mitigate the impact of climate change, support the livelihoods of 40% of the extreme rural poor –250 million people–, and sustain more than three quarters (¾) of the global biodiversity.
Fire responders have limited resources to fight large scale forest fires. And they are using inefficient methods and tools to plan and allocate those scarce resources. The forecasting of fire behavior and spreading is carried out by using old methods and cumbersome tools that are not taking advantage of the last few years of advances in UAVs, machine learning and small-factor computers.
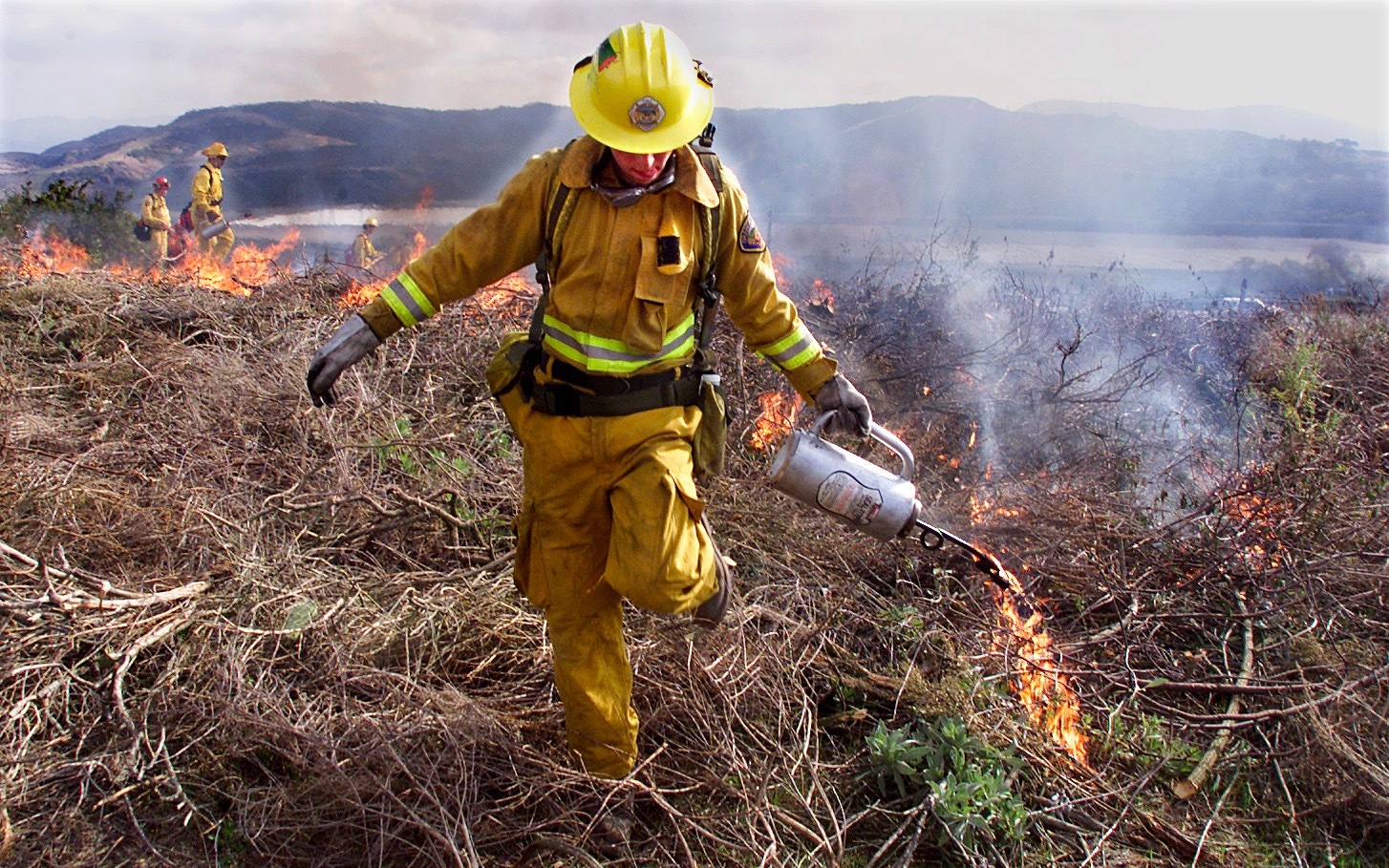
Responders in the global south make almost no use of technology such as UAVs for exactly the two reasons this challenge states:
1. Lack of a use-case specific tool that simplifies the user experience and allows non-technical people to apply it, and
2. The ubiquitous requirement of connectivity to process imagery and run simulations or models.
In addition to that, the few forest fire modeling software solutions available (like Flammap) require a deep knowledge of GIS tools, a lot of manual work to prepare inputs, and no integration with local weather stations.
How it works
There are 3 key elements that make this solution different to anything else out there:
1. Multispectral imagery updated often with the use of UAVs
2. Machine learning algorithms to classify the terrain
3. A small, purpose built, portable weather station
All of these components combined, enable us to build an accurate, local and almost real-time forecast of wildfire spreading. By combining multispectral aerial images and other variables collected by drones and portable weather stations, like temperature readings, humidity, wind speed and direction, ForestFires.tools can create a more accurate, local, and up-to-date model, that allows firefighters to better understand and respond to forest fires. Additionally, using a machine learning algorithm to classify the types of vegetation in the fire surroundings, we can predict the final shape and area of a fire over specific periods of time, as well as the speed and temperature at which the fire will burn in different areas.
The data sources that are gathered by the UAVs are then processed by an inexpensive, portable computer that doesn’t require an Internet connection to prepare a fast fire model capable of forecasting the spread of the fire. Combining all those data points, we can model and forecast the way the fire will spread at a more local level and in shorter cycles, providing responders with more accurate and real-time information for decision-making. This gives firefighters autonomy on the field, without losing access to accurate, timely information.
Results
These wildfire behavior forecasting tools deliver an hour-by-hour machine learning assisted forecast of forest fires shape and area. The outcome is precise and timely knowledge in the hands of firefighters, which significantly improves their ability to allocate resources and make decisions, as efficiently as possible.
The final impact is that forest fires will be contained sooner, burning less forest area, emitting less CO2, contributing less to climate change, sustaining a larger amount of wildlife and biodiversity in general, and enabling the livelihoods of those depending on those forests.
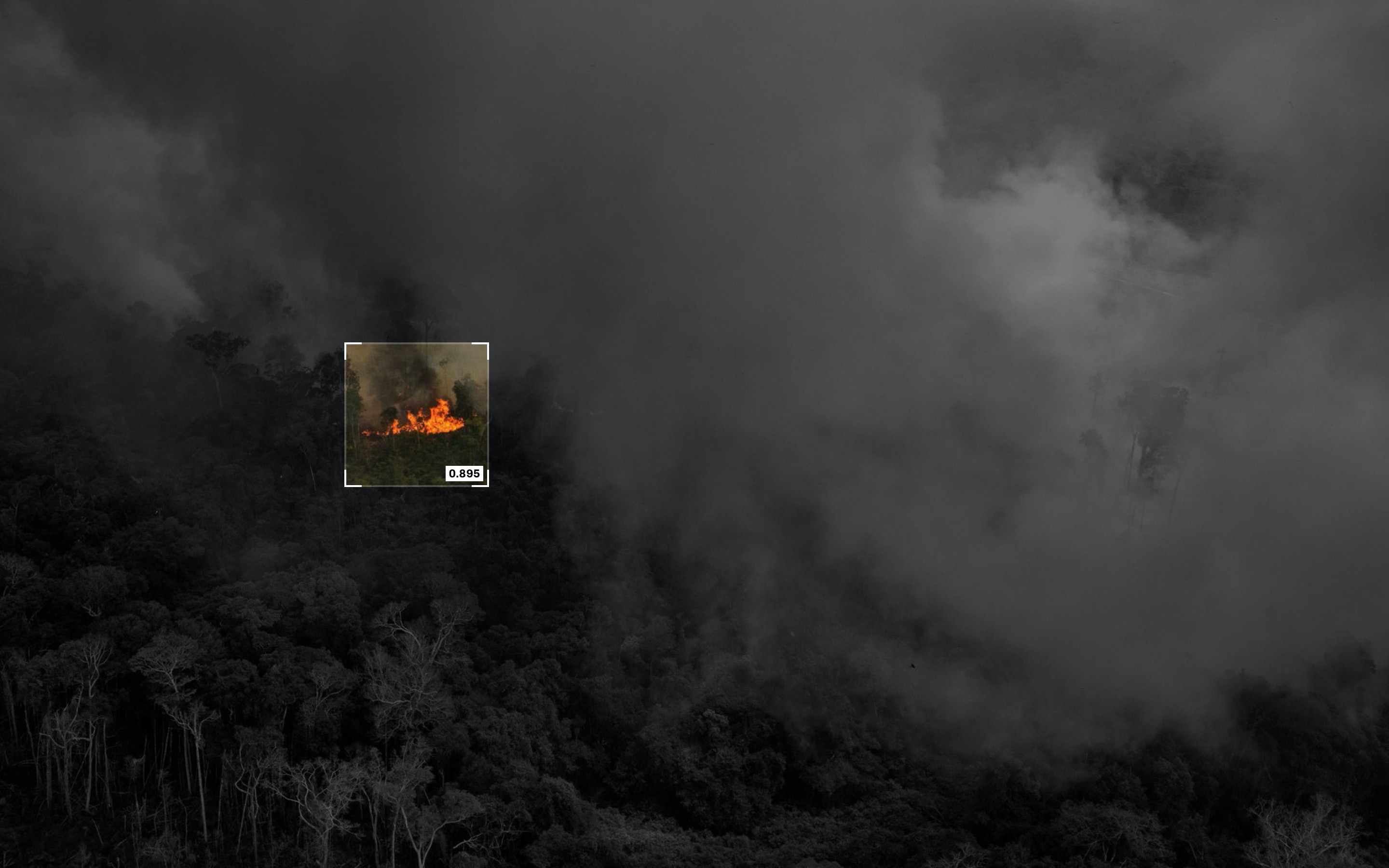
Impact
The potential impact of such a tool is a more focused and efficient use of resources during forest fires response efforts. If responders have a better understanding of the likelihood of the fire spreading in certain directions and at certain speeds, efforts can be better focused, and evacuations –if necessary– could be planned with more time.
Given that forests provide widespread and uniform benefits to humans, animals and plants, and since the final goal of this tool is to reduce the negative impact of forest fires, it is tempting to say that all of the living species in the world benefit from it. A more direct group of beneficiaries would be the ~250 million people living in extreme poverty in rural areas, whose livelihoods depend on forests.
Focusing only on directly affected populations, the most relevant data we could find is that 5 million people have been affected by wildfires between 1984 and 2013.
Next steps
ForestFires.tools was developed as a concept for the Unusual Solutions Competition, and has not yet been deployed in a real setting. However, it is as close to completion as possible without financing. If you’re interested in making it a reality, just go to ForestFires.tools and get in touch.